Imagine diagnosing critical diseases in seconds. Thanks to cloud GPUs, that’s becoming a reality. Medical imaging generates massive amounts of data, and cloud-based GPUs process it quickly, accurately, and remotely, transforming healthcare diagnostics.
But why cloud GPUs? Traditional systems can’t keep up with the demands of CT scans, MRI, and real-time imaging. Cloud GPUs offer speed, scalability, and cost-efficiency without the need for expensive on-site hardware.
What’s more, AI-powered diagnostics are leaping forward, using cloud GPUs to run deep learning models that detect diseases faster and more accurately. Healthcare providers worldwide are adopting these solutions for quicker, more reliable diagnostics.
Ready to discover how cloud GPUs are reshaping medical imaging? Let’s dive in!
Table of Contents
How Cloud GPUs are Enhancing Medical Imaging?
Cloud GPUs revolutionize medical imaging by delivering unmatched speed and scalability. Traditional systems struggle to handle the enormous data generated by modern imaging techniques, but cloud GPUs make real-time diagnostics possible—no matter the location of healthcare providers.
This shift is not only about speed; it’s transforming the way professionals analyze and store data, leading to more efficient workflows and better patient outcomes.
But how exactly do cloud GPUs achieve this? Let’s break it down.
The Role of Cloud Computing in Healthcare
Cloud computing plays a pivotal role in modern healthcare, reshaping how medical data is processed, stored, and analyzed. With the ever-growing volume of healthcare data generated through medical imaging, electronic health records (EHRs), and other diagnostic processes, cloud infrastructure offers a scalable, cost-efficient, and secure solution for healthcare providers.
Here’s why cloud computing is essential for medical imaging and diagnostics:
Cloud infrastructure for medical imaging allows hospitals to process and store massive datasets without needing costly, high-maintenance on-premise hardware. This results in cost savings of up to 50% for hospitals that transition from traditional servers to cloud-based solutions.
According to a report from MarketsandMarkets, the healthcare cloud computing market is expected to grow from $39.4 billion in 2020 to $89.4 billion by 2027, with a significant portion driven by medical imaging applications.
Cloud GPUs for medical research improve data processing speed and efficiency, enabling healthcare professionals to analyze complex datasets in real-time. This is particularly crucial for AI-based diagnostics, where high volumes of imaging data (such as CT, MRI, and PET scans) need to be processed quickly to generate actionable insights.
On average, cloud GPUs can reduce processing time for large datasets by 40% to 60%, allowing healthcare providers to diagnose and treat patients faster than ever before.
Enhanced collaboration and accessibility: Cloud platforms enable secure, real-time collaboration between healthcare professionals, regardless of location. Radiologists and physicians can access the same imaging data, consult with specialists, and provide diagnoses remotely, enhancing the efficiency and accuracy of healthcare services.
Major Platforms Utilising Cloud-Based GPUs
NVIDIA Clara Platform
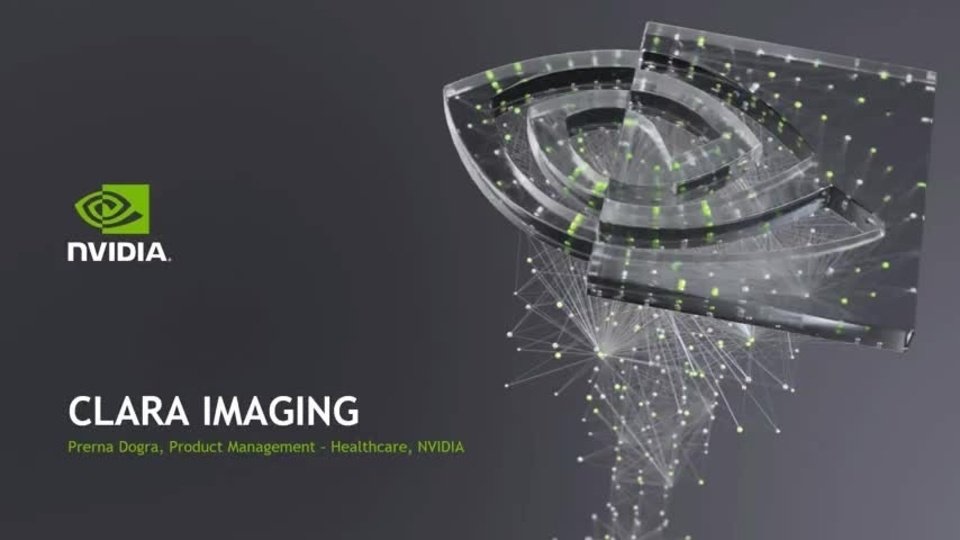
NVIDIA Clara is a cloud-based solution that uses GPU-accelerated computing to advance diagnostics in medical imaging. Designed specifically for healthcare, the Clara Platform leverages AI-powered diagnostics and high-performance computing to analyze and process large medical imaging datasets.
This enables healthcare providers to perform complex analyses with speed and accuracy that would be difficult to achieve with traditional systems.
- AI-Powered Diagnostics: NVIDIA Clara incorporates deep learning algorithms to interpret a range of imaging modalities, including CT scans, MRI, X-rays, and ultrasound. By using these AI models, the platform can help detect subtle patterns in images that may indicate serious health conditions, such as cancer, cardiovascular issues, and neurological disorders. For instance, Clara’s AI can highlight abnormal tissue areas or detect potential blockages in cardiac scans, enabling radiologists and doctors to identify issues earlier and with greater confidence.
- Accelerating Diagnosis: Clara’s GPU-based processing accelerates the analysis of large imaging datasets, significantly reducing the time required to process images. In traditional imaging setups, analyzing high-resolution 3D scans can take several minutes or even hours, but with Clara’s cloud-based GPUs, processing times can be reduced to a matter of seconds or minutes. This is particularly valuable in emergency settings, where fast and accurate imaging is crucial to patient outcomes.
- Collaborations with Healthcare Institutions: NVIDIA Clara is used by top medical institutions worldwide, including Mass General Brigham and Mayo Clinic, to support advanced diagnostic workflows. Through collaborations, Clara has been integrated into clinical settings to enable automated image analysis, support clinical decision-making, and provide research tools for developing new diagnostic algorithms.
- Enhanced Precision in Disease Detection: The platform’s AI models are trained on diverse datasets, which helps improve accuracy in identifying diseases across various demographics and clinical conditions. For example, Clara’s AI tools are known to improve early detection of lung cancer and brain aneurysms, allowing for better patient management and prognosis.
In summary, NVIDIA Clara Platform is transforming medical imaging by combining cloud-based GPUs with AI-powered diagnostics to deliver faster, more accurate results. This platform’s speed, scalability, and accuracy make it an invaluable tool for healthcare providers looking to enhance patient care through data-driven insights.
NVIDIA Clara Guardian
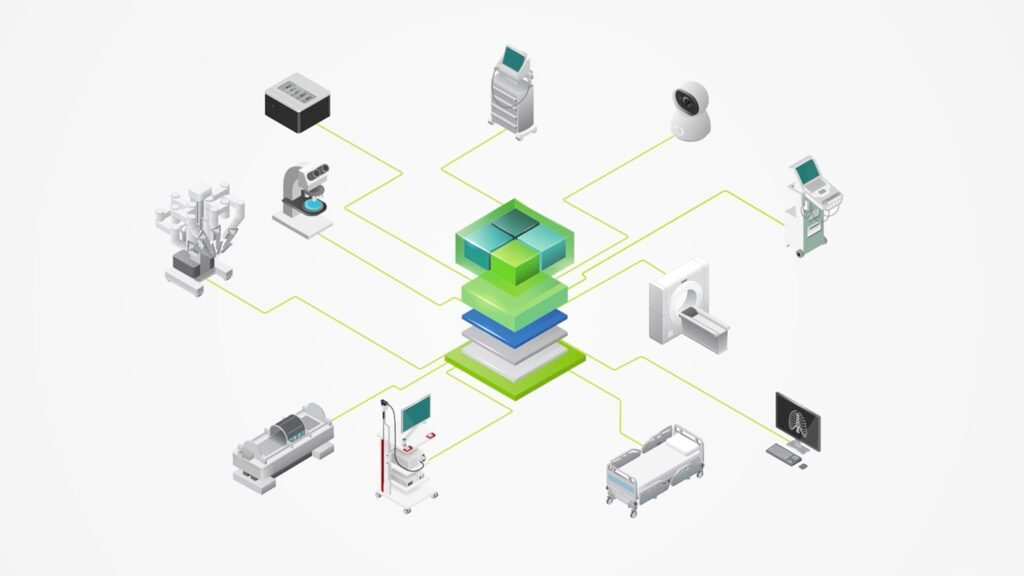
NVIDIA Clara Guardian is an extension of the Clara Platform that integrates AI models into healthcare facilities to improve patient monitoring and clinical workflows.
This platform is specifically designed for smart hospital environments, providing real-time video analytics and sensor integration to track patient activity, monitor vital signs, and detect potential safety risks.
- Real-Time Patient Monitoring: Clara Guardian uses AI-enhanced video analytics to observe patient behaviors and activities. For example, cameras equipped with Clara Guardian can monitor patients for signs of distress, unassisted movement, or falls, which is particularly valuable in intensive care units (ICUs) and elderly care facilities. This capability helps healthcare providers respond promptly to patient needs and reduce the risk of adverse events.
- Smart Sensor Integration: The platform integrates smart sensors to collect a wide range of patient data, including heart rate, respiratory rate, and body temperature. These sensors operate continuously, allowing for real-time patient monitoring without the need for invasive procedures or manual data collection. In combination with cloud-based GPUs, Clara Guardian can process this data instantly, alerting healthcare providers to any significant changes in patient condition.
- Enhanced Clinical Workflows: By automating routine tasks and providing real-time alerts, Clara Guardian enhances clinical workflows and improves efficiency in hospital settings. For instance, Clara Guardian can be used to monitor hand hygiene compliance among staff, a critical factor in infection control within hospitals. This automated monitoring ensures that healthcare workers adhere to protocols, reducing the risk of hospital-acquired infections.
- Application in Pandemic Response: During the COVID-19 pandemic, Clara Guardian was adapted to support contactless monitoring and social distancing compliance in healthcare facilities. By using AI-powered sensors and video analytics, the platform helped hospitals manage patient and staff safety protocols, reducing the spread of infection and ensuring efficient patient care.
- Data Privacy and Compliance: Clara Guardian is designed with data privacy in mind. It follows stringent security standards to ensure compliance with HIPAA and other healthcare regulations, which is essential for the safe handling of patient data and for maintaining trust with patients and healthcare providers.
In summary, NVIDIA Clara Guardian transforms patient monitoring in healthcare by integrating real-time video analytics and smart sensor technology into clinical environments. Its ability to provide continuous, non-invasive monitoring improves patient safety, supports more responsive care, and allows healthcare staff to focus on critical patient needs.
Features of NVIDIA Clara Platform vs. NVIDIA Clara Guardian
Feature | NVIDIA Clara Platform | NVIDIA Clara Guardian |
Primary Function | AI-powered medical imaging analysis | Real-time patient monitoring and workflow automation |
Use Cases | Diagnostics for conditions like cancer, cardiac issues, neurological disorders | Monitoring patient behaviors, hygiene compliance, and safety in hospitals |
AI Capabilities | Deep learning for detecting diseases | AI-enhanced video analytics and smart sensors |
Data Processing | High-speed GPU processing for large imaging datasets | Real-time data processing for patient monitoring |
Example Applications | Used by Mayo Clinic for MRI analysis | Used during COVID-19 for contactless monitoring |
Regulatory Compliance | HIPAA compliant | HIPAA compliant with focus on patient data privacy |
This table can highlight the distinct features and use cases of NVIDIA Clara Platform and NVIDIA Clara Guardian to help readers quickly understand their differences.
NIH Cloud Platforms
These platforms enable large-scale medical data processing, facilitating collaborative research and diagnosis. Researchers and physicians can seamlessly process and analyze massive datasets like genomic data, MRI scans, and CT imaging through the cloud.
This accelerates research in fields such as genomics, cancer research, and population health.
In one NIH study, the cloud platform processed over 1 million MRI images in half the time it would have used traditional, on-premise infrastructure, highlighting the time-saving benefits of cloud computing.
AWS HealthLake:
This service enables healthcare organizations to store, transform, and query EHR data and imaging datasets. By structuring health data and leveraging machine learning, AWS HealthLake helps healthcare professionals quickly uncover trends and patterns in patient data, improving diagnosis and treatment decisions.
AWS HealthLake is used by major hospitals to analyze large volumes of diagnostic imaging data, cutting down on time-intensive manual processes and providing doctors with predictive insights into patient conditions.
Why Medical Imaging Needs GPUs?
Medical imaging, such as CT scans, MRIs, and DICOM files, produces enormous amounts of data that need to be processed in real time for accurate diagnostics.
Traditional systems often struggle to handle the sheer volume of data, but GPUs have become indispensable in modern medical imaging due to their ability to accelerate image processing.
Here’s why GPUs are critical for medical imaging:
Immense computational power
Medical image analysis requires vast computational resources to process high-resolution images quickly and efficiently. GPUs significantly outperform traditional CPUs in handling the complex computations needed for image rendering and analysis.
Each MRI scan can produce hundreds of images, requiring advanced GPU-powered diagnostic tools to analyze and interpret these images in real-time.
Faster image rendering
With GPU healthcare acceleration, medical images can be processed in seconds rather than minutes. This reduction in rendering time allows doctors to gain actionable insights more quickly, enabling faster decision-making in critical situations.
In emergency settings, where every second counts, cloud GPUs can accelerate MRI and CT scan analysis by up to 60%, giving doctors the information they need to act swiftly.
Cloud-based scalability
Unlike on-premise systems, cloud GPUs provide scalable computing power that adjusts to demand. Whether handling routine diagnostic images or managing a surge in emergency cases, cloud GPUs can easily scale up to meet processing needs.
According to the NIH Cloud Supplement (2024), cloud-based GPUs reduce processing times for large datasets by up to 60%, enabling more healthcare providers to access high-speed diagnostics, especially in time-sensitive medical cases.
What’s the real impact? Faster diagnoses, more accurate results, and ultimately, better patient outcomes.
By integrating GPUs into their medical imaging workflows, healthcare providers can deliver improved care with greater speed and precision.
Cloud GPUs vs. On-Premise GPUs
When it comes to medical imaging, healthcare providers often ask: “Which is better, cloud GPUs or on-premise GPUs?”
Both options have their merits, but cloud-based GPUs offer several advantages that make them a better fit for modern healthcare needs.
Let’s break down how cloud GPUs outperform on-premise GPUs in key areas like scalability, cost-efficiency, and remote access.
1. Scalability
Cloud GPUs provide dynamic scalability, meaning healthcare facilities can easily scale up or down their GPU resources based on real-time demand. This is especially useful during surges in medical imaging needs, such as during public health emergencies, when the ability to process more imaging data without additional hardware is crucial.
For example, During a pandemic, hospitals using cloud-based GPU systems can instantly increase their computing power to process more MRI and CT scans, ensuring timely diagnostics without the delays associated with upgrading on-premise systems.
This allows for quick adaptation without any delays or need for hardware expansion.
On-premise systems lack scalability. Expanding the capacity requires a large upfront investment in additional hardware and infrastructure, which can be time-consuming and expensive.
As patient volumes grow, on-premise solutions may face bottlenecks, reducing diagnostic efficiency.
2. Cost-Efficiency
Cloud-based GPUs are a cost-efficient solution for healthcare facilities, as they eliminate the need for heavy upfront investment in physical hardware. Instead, providers pay for GPU resources based on actual usage, making this model especially attractive for smaller clinics and hospitals with fluctuating workloads.
Healthcare institutions adopting cloud GPU systems have reported cost savings of up to 40% on infrastructure and maintenance, thanks to the pay-as-you-go model and reduced operational expenses.
On-Premise GPUs, in contrast, involve high upfront costs for buying hardware, setting up cooling systems, and hiring IT staff for ongoing maintenance. Over time, these costs accumulate, making on-premise systems less viable for smaller healthcare facilities.
3. Remote Access and Collaboration
Cloud-based GPU systems allow healthcare providers to access medical imaging data from anywhere. This is particularly beneficial for remote diagnostics and collaboration between specialists in different locations. Radiologists, physicians, and technicians can easily work together in real-time, leading to faster decision-making and improved patient outcomes.
A radiologist in New York can consult with a specialist in California in real-time using cloud GPUs for healthcare, reviewing the same MRI or CT scan without needing to be in the same physical location.
On-premise GPUs restrict remote access because they are confined to a physical network. Setting up remote access for on-premise systems typically requires VPNs, which can introduce latency and connectivity issues, slowing down diagnostics.
4. Maintenance and Updates
Cloud-based GPU systems are automatically updated, meaning healthcare providers receive the latest features, security patches, and performance improvements without manual intervention. This ensures that the system is always operating at peak efficiency without downtime for updates or maintenance.
On-premise GPUs, however, require frequent manual maintenance, including updates and security patches. This can cause workflow disruptions, and as hardware becomes outdated, providers may face costly upgrades to maintain optimal performance.
5. Real-World Impact of Cloud GPUs
Using Cloud GPUs in Healthcare has demonstrated real-world benefits in medical imaging workflows. Hospitals utilizing GPU-accelerated PACS systems through the cloud have seen smoother operations, with diagnostic times reduced by up to 30%. This leads to faster treatment decisions and improved patient outcomes.
With cloud-based PACS integration, healthcare professionals can access imaging data instantly, without delays caused by on-premise systems. This ensures timely diagnoses, especially in emergency care settings.
While on-premise GPUs provide control over hardware and data, their limitations in scalability, cost-efficiency, and remote access make them less ideal for modern healthcare needs. Cloud GPUs, on the other hand, offer flexible, scalable, and cost-effective solutions that cater to the growing demands of medical diagnostics. They enable real-time collaboration, reduce diagnostic times, and lower operational costs.
For healthcare providers seeking to enhance performance and reduce costs, transitioning to cloud-based GPUs is a smart solution for meeting the future demands of medical imaging.
Benefits of Cloud GPUs in Medical Imaging Applications
Cloud GPUs are a game-changer for medical imaging, offering healthcare providers the tools they need to process complex data quickly and efficiently.
Let’s dive into the key benefits they bring to medical imaging, from speed and scalability to cost savings and AI integration.
Faster Image Processing
Cloud GPUs revolutionize medical imaging by drastically reducing image processing time, and improving diagnostic speed and accuracy. Whether it’s real-time diagnostic imaging or handling large datasets, cloud GPUs enable quicker results and more efficient care.
The NVIDIA NVImageCodec is a perfect example, decoding medical images rapidly, and providing real-time feedback that enhances diagnostic accuracy. This tool reduces image processing times by up to 50%, ensuring patients receive timely care.
Scalability and Flexibility for Healthcare Providers
Cloud GPUs provide unmatched scalability, allowing healthcare providers to adjust their computing power as needed. This flexibility eliminates the need for costly hardware investments, ensuring that hospitals can scale resources up or down based on demand.
Healthcare facilities using cloud-based infrastructures, such as Amazon Web Services (AWS), benefit from scalable GPU usage that adapts to their requirements, ensuring smooth and efficient operations during high-demand periods.
This results in operational cost savings of up to 40% for facilities that handle high-volume imaging data during peak times.
By embracing this scalable approach, hospitals can optimize their operations without the constraints of traditional on-premise systems.
Enhanced AI and Deep Learning in Diagnostics
AI-driven medical platforms are changing the face of healthcare, and cloud GPUs are the backbone of this revolution. These platforms run deep learning models that detect diseases earlier and with more accuracy than traditional methods.
The NVIDIA Clara platform helps healthcare professionals use AI to detect early signs of diseases such as cancer.
In one case study, AI models running on cloud GPUs improved early cancer detection rates by 20%, leading to better patient outcomes.
Cost-Effectiveness and Efficiency
Cloud GPUs eliminate the high costs associated with maintaining in-house GPU infrastructure. Instead, healthcare providers can opt for pay-as-you-go models, allowing them to manage expenses more effectively.
The NIH Cloud Supplement reports that cloud-based solutions can reduce operational costs by up to 50%, particularly for smaller clinics and healthcare providers.
Cloud GPUs also improve efficiency by allowing healthcare staff to focus on patient care rather than managing complex hardware systems.
Key Applications of Cloud GPUs in Medical Imaging
Cloud GPUs are unlocking new possibilities in medical imaging, enabling faster, more accurate diagnostics across various healthcare applications. From high-resolution imaging to AI-assisted diagnostics, cloud GPUs are transforming how healthcare providers process and analyze medical images.
These advances are helping to improve patient care by increasing the speed and precision of medical image analysis.
Let’s explore the most impactful uses of this technology in the healthcare industry.
Computed Tomography (CT) and MRI Processing
Cloud GPUs play an essential role in processing the massive datasets generated by Computed Tomography (CT) and Magnetic Resonance Imaging (MRI) scans. These scans produce high-resolution 3D images that require substantial computational power to analyze effectively, often in real-time.
Real-time image rendering is critical in emergencies where rapid diagnosis can save lives. GPUs provide the necessary speed and efficiency to render these complex images quickly and accurately, reducing the time needed for image analysis and allowing doctors to make faster decisions.
In many hospitals, NVIDIA GPUs are used to accelerate CT and MRI image rendering, enabling radiologists to process large imaging datasets more efficiently. According to a study by Cedars-Sinai Medical Center, the use of NVIDIA Clara GPUs reduced image processing times for MRI scans by up to 30%, leading to faster diagnosis and improved patient outcomes.
Studies show that GPU-powered diagnostic tools in cloud computing environments can improve medical imaging speeds by 60%, ensuring quicker diagnostics in critical care settings.
By leveraging cloud GPUs, healthcare providers can enhance the speed and clarity of CT and MRI imaging, allowing for more precise diagnoses and faster intervention, which ultimately improves patient care.
AI-Assisted Image Diagnostics
Cloud GPUs are crucial for running AI-powered healthcare diagnostics, where deep learning models analyze medical images to identify diseases such as cancer, cardiac conditions, and neurological disorders.
These AI models require immense computational power to process vast amounts of data and recognize patterns that may be invisible to the human eye.
NVIDIA Clara Platform, This platform is widely used in hospitals to develop AI-driven medical platforms that support faster and more accurate diagnoses. By utilizing deep learning models, these tools help detect early signs of serious diseases, leading to better treatment outcomes for patients.
At Mount Sinai Hospital, the use of AI-powered tools combined with cloud GPUs accelerated the detection of lung cancer in CT scans by 50%, allowing doctors to diagnose and treat patients at earlier stages, significantly improving survival rates.
AI-powered diagnostics running on cloud GPUs can analyze medical images up to 10 times faster than traditional systems, offering early-stage intervention that leads to more favorable patient outcomes.
With the power of cloud GPUs, healthcare professionals can harness AI to enhance their diagnostic capabilities, offering more precise and timely interventions.
Real-Time Diagnostics in Radiology
Real-time diagnostics represent a vital application of cloud GPUs in radiology. By enabling real-time medical image rendering, cloud GPUs allow radiologists to analyze images remotely and provide instant feedback, regardless of their location. This capability is particularly beneficial for emergency care and rural healthcare settings where immediate access to specialists is often limited.
Cloud-based radiology platforms powered by GPUs enable radiologists to provide real-time diagnostic support to remote or underserved areas. For instance, Partners HealthCare in Boston uses cloud GPUs to provide remote radiology services to rural clinics across the U.S., reducing diagnostic delays and improving patient care.
According to Frost & Sullivan, hospitals using cloud-based radiology platforms powered by GPUs saw a 35% reduction in diagnostic time for emergency cases, which dramatically improved patient outcomes.
The flexibility of cloud GPUs enables hospitals and clinics to maintain continuous access to diagnostic tools, even in remote or crises, ensuring that patients receive accurate diagnoses without the need for on-site specialists.
By integrating cloud GPUs into radiology workflows, healthcare facilities can ensure faster, more accurate diagnoses, providing better outcomes for patients regardless of location or situation.
Overcoming Challenges in Adopting Cloud GPUs for Medical Imaging
Adopting cloud GPUs in medical imaging offers tremendous benefits, from faster diagnostics to enhanced scalability, but it also presents certain challenges. From ensuring data security to managing latency and costs for smaller facilities, these hurdles must be carefully addressed to ensure successful integration.
Here’s a look at the most common challenges and the solutions that can make cloud GPU adoption smoother and more effective for healthcare providers.
Data Privacy and Security Concerns
One of the biggest concerns for healthcare providers is ensuring data privacy and security when using cloud-based systems. Given the sensitive nature of medical data, any breach could have serious implications. Fortunately, robust security protocols and strict regulatory compliance measures can help mitigate these risks.
To safeguard healthcare data security, most cloud platforms follow stringent regulatory standards, such as HIPAA compliance in the U.S. Platforms like NIH Cloud Platforms offer secure infrastructure with advanced encryption, multi-factor authentication, and data access controls to maintain confidentiality and prevent unauthorized access.
According to a report by HIPAA Journal, over 85% of healthcare providers using cloud solutions have successfully met or exceeded HIPAA security standards, highlighting the effectiveness of advanced security measures.
By prioritizing robust security, healthcare providers can adopt cloud GPUs without compromising data privacy and remain compliant with healthcare regulations.
Latency and Network Issues
Latency is another critical challenge in adopting cloud GPUs for latency-sensitive imaging applications. Medical imaging requires real-time processing, and any delay could affect diagnostic accuracy and response times. Minimizing latency is essential, particularly for remote diagnostics or emergency care settings where timing is crucial.
Cloud providers, such as Google Cloud, leverage edge computing to reduce latency by processing data closer to the source. Edge nodes allow medical facilities to analyze imaging data with minimal delay, ensuring that critical diagnostics are not compromised by network issues.
Hospitals using Google Cloud’s edge computing solutions reported up to 40% reductions in latency for real-time imaging diagnostics, ensuring that critical diagnostic information reaches radiologists and physicians quickly.
By leveraging advanced cloud technologies, healthcare providers can ensure that latency does not interfere with critical diagnostic processes, even in remote or underserved areas.
Cost Management for Smaller Healthcare Facilities
For smaller healthcare facilities, managing the costs of adopting cloud GPUs can be challenging. Unlike larger institutions, smaller clinics may have budget constraints, making it harder to invest in new technology. However, cloud solutions offer flexible scalability and pricing models that make cost management feasible.
With scalable healthcare computing systems, clinics can choose pay-as-you-go models from providers like AWS and Microsoft Azure. This way, they only pay for the GPU resources they use, making cloud GPUs more accessible and affordable.
Research from MarketsandMarkets indicates that pay-as-you-go models have reduced cloud adoption costs for smaller healthcare facilities by 30% on average, enabling them to leverage high-performance computing without straining their budgets.
By choosing cost-effective cloud options, smaller healthcare providers can enjoy the advantages of cloud GPUs without overextending their budgets, ensuring that quality care is accessible to all patients.
How to Select the Best Cloud GPU Solution for Medical Imaging
Choosing the right cloud GPU solution for medical imaging is critical to ensuring seamless workflows and accurate diagnostics. With so many options available, it’s essential to evaluate your facility’s specific needs and compare providers based on performance, scalability, and cost-effectiveness.
Here are the key factors to consider when choosing a cloud GPU solution for healthcare.
Understanding Your Imaging Needs
The first step in selecting the right cloud GPU solution is to assess your facility’s imaging needs. The specific type of medical imaging your facility performs—whether CT scans, MRIs, or general diagnostic imaging—will determine the required computational power. For example, facilities with high MRI workloads may need more powerful GPU solutions for efficient processing.
Hospitals that handle intensive MRI imaging often select cloud GPU solutions with higher computational power to optimize processing speeds and ensure GPU healthcare optimization tailored to their specific needs.
Mayo Clinic uses NVIDIA Clara to support MRI diagnostics, reducing imaging times by up to 25% and enabling radiologists to manage large volumes of MRI data efficiently.
By aligning your cloud GPU solution with your facility’s imaging requirements, you can optimize both efficiency and performance.
Comparing Cloud Providers for Medical Imaging
Different cloud providers offer unique features, pricing models, and support levels for cloud GPUs in healthcare. Leading providers like Amazon Web Services (AWS), Google Cloud, and Microsoft Azure each have dedicated cloud medical platforms, but their offerings vary in scalability, cost, and data security.
To find the best fit for your needs, evaluate providers based on pricing models, data security protocols, scalability options, and AI integration. Many healthcare facilities use detailed feature comparison charts to streamline the decision-making process.
AWS HealthLake offers integrated data management and AI tools, which help hospitals process and analyze large imaging datasets while maintaining strict compliance with HIPAA and HITECH standards. In comparison, Google Cloud Healthcare API focuses on interoperability, allowing healthcare providers to easily share imaging data with external specialists securely.
By comparing cloud medical platforms and evaluating key aspects, you can select a cloud GPU solution that provides the right balance of power, flexibility, and value for your facility.
Integrating PACS Systems with Cloud GPUs
Integrating PACS (Picture Archiving and Communication Systems) with cloud GPUs can greatly enhance workflow efficiency, reducing manual tasks and improving access to diagnostic data. By automating the storage and retrieval of medical images, cloud-based PACS systems ensure that critical imaging data is always available when needed.
Hospitals using cloud-based PACS systems report a 30% improvement in workflow efficiency and faster diagnostic times, as the system automates image retrieval and storage.
At Cleveland Clinic, integrating cloud GPUs with PACS allowed radiologists to access patient imaging data remotely, cutting diagnostic delays by 20% and improving overall patient care quality.
Integrating PACS with cloud GPUs not only streamlines workflows but also boosts the quality of patient diagnostics by providing instant access to critical imaging data. This makes it a valuable addition to any healthcare facility aiming to enhance operational efficiency and diagnostic accuracy.
Future of Cloud GPUs in Medical Imaging
The future of cloud GPUs in medical imaging is set to be transformative. Advancements in AI-driven diagnostics, real-time imaging, and enhanced data security will revolutionize how healthcare providers diagnose and treat patients.
As these technologies evolve, cloud GPUs are poised to become indispensable tools in modern healthcare, enabling faster, more accurate, and accessible diagnostics.
AI-Driven Innovations in Diagnostics
Artificial intelligence (AI) and machine learning (ML) are pushing the boundaries of what’s possible in medical imaging diagnostics. With AI-driven healthcare models, cloud GPUs will support increasingly sophisticated tools that allow healthcare professionals to detect diseases at earlier stages with improved accuracy and speed.
NVIDIA Clara is at the forefront of these advancements, partnering with institutions like Mass General Brigham to develop cutting-edge AI diagnostic tools. These tools analyze complex imaging data faster and more accurately than traditional methods, reducing the time needed for analysis and enabling quicker interventions.
NVIDIA Clara’s AI models have demonstrated improved diagnostic accuracy in detecting diseases like lung cancer, increasing early detection rates by up to 25% in trials.
According to Allied Market Research, the global AI in the healthcare market is projected to reach $194.4 billion by 2030, with medical imaging as one of the largest segments. This growth is directly linked to advancements in cloud GPU-powered AI diagnostics, which make it possible to analyze larger datasets with greater precision.
As AI continues to evolve, cloud GPUs will play a critical role in expanding the reach and capabilities of AI-powered diagnostics, enabling faster and more proactive healthcare.
Increased Adoption of Real-Time Imaging Solutions
The demand for real-time medical imaging is expected to rise sharply as healthcare facilities increasingly recognize the benefits of cloud-based solutions. With real-time imaging, healthcare providers can deliver rapid diagnostics, especially valuable in emergencies or rural areas with limited access to specialized care.
The adoption of real-time medical image rendering and other advanced diagnostic tools is expected to continue rising as more hospitals and clinics recognize the benefits of cloud GPUs. According to recent projections, AI has the highest projected adoption rate in healthcare technologies, making cloud GPU platforms critical for supporting these advancements. Other technologies like Remote Monitoring and IoT are also seeing rapid growth in adoption, further supporting the integration of cloud solutions in healthcare workflows.
According to the NIH Cloud Supplement (2024), the use of cloud GPUs for real-time diagnostics is anticipated to grow by over 50% in the next five years. This shift is driven by healthcare providers’ need to improve patient care through more timely and accessible solutions, especially in remote settings where real-time imaging can bridge the gap in specialist access.
In rural healthcare centers, real-time imaging powered by cloud GPUs allows radiologists to instantly share and analyze images with specialists in urban hospitals, reducing the time needed for diagnosis and enabling quicker treatment decisions.
This has proven particularly beneficial for conditions like stroke, where rapid diagnosis is critical to improving outcomes.
As more cloud GPU-powered platforms enter the market, the flexibility and speed offered by real-time imaging will become a standard feature, helping healthcare providers meet the rising demand for fast, accurate diagnostics and enhanced patient care.
Advancements in Cloud Security for Healthcare
As healthcare providers increasingly adopt cloud GPUs, the need for more advanced healthcare data security measures becomes critical. Future innovations in cloud data security will focus on making systems more secure and reliable, protecting sensitive medical data from breaches and unauthorized access.
Upcoming advancements will include enhanced encryption, multi-factor authentication, blockchain-based data integrity checks, and automated threat detection systems. These innovations protect healthcare data at every level, from storage to transmission.
AWS HealthLake uses advanced encryption and identity management to ensure data security, enabling healthcare providers to securely store and access patient information in compliance with HIPAA and HITECH. This level of security is becoming the industry standard for cloud-based healthcare systems.
Adhering to regulations like HIPAA in the U.S. and GDPR in the EU is critical as cloud data security in healthcare advances. Compliance with these regulations not only protects data but also builds patient trust, as individuals become increasingly aware of data privacy concerns.
Enhanced data encryption and biometric access controls are expected to be incorporated into cloud-based medical systems in the next decade. Additionally, AI-driven security algorithms will monitor network activity to detect potential threats in real-time, ensuring that data is both accessible and secure.
By prioritizing these advanced security measures, future cloud GPU solutions will provide healthcare providers with greater peace of mind, making data security a cornerstone of cloud-based healthcare systems.
Conclusion
With the continued evolution of AI, real-time imaging, and data security, cloud GPUs are shaping up to be vital assets in medical imaging.
These advancements will not only improve the speed and accuracy of diagnostics but also enhance patient outcomes and provide healthcare providers with more reliable, flexible, and secure tools for the future.
The future of cloud GPUs in healthcare is promising, and those who adopt these solutions are set to lead the charge in delivering better, faster, and safer healthcare.